本文已被:浏览 1233次 下载 1174次 |
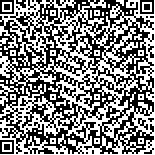 码上扫一扫! |
|
基于机器学习的青岛市区近岸海雾集成预报方法 |
高松1,2, 徐江玲1,2, 刘桂艳1,2, 毕凡1,2, 张薇1,2, 白涛1,2
|
1.国家海洋局北海预报中心, 山东 青岛 266061;2.山东省海洋生态环境与防灾减灾重点实验室, 山东 青岛 266061
|
|
摘要: |
利用2014—2017年青岛小麦岛海洋站观测资料,采用机器学习方法建立了青岛市区近岸海雾集成预报模型,通过主成分分析方法对预报因子进行了优选。结果表明:采用能见度、风向、风速、气压、露点、气温、海温、气温露点差、气海温差、相对湿度、云量、气温24h变温12个预报因子建立的海雾集成预报模型,对2018年海雾预报的TS评分约为0.64,海雾预报正确率约为0.783,具有较好的预报能力,为海雾预报提供了新的方法。 |
关键词: 海雾 机器学习 集成预报 青岛沿海 |
DOI:10.11759/hykx20200310004 |
分类号:P732 |
基金项目:国家重点研发计划资助(2016YFC1401409) |
|
Ensemble forecast of sea fog in Qingdao coastal area based on machine learning |
GAO Song1,2, XU Jiang-ling1,2, LIU Gui-yan1,2, BI Fan1,2, ZHANG Wei1,2, BAI Tao1,2
|
1.North Sea Marine Forecast Center of State Oceanic Administration, Qingdao 266061, China;2.Shandong Provincial Key Laboratory of Marine Ecological Environment and Disaster Prevention and Mitigation, Qingdao 266061, China
|
Abstract: |
Based on the 2014-2017 observation data of the Xiaomaidao marine station in Qingdao Shelf, ensemble forecast models of sea fog in the Qingdao coastal area were established using machine learning. The principal component analysis method was adopted to optimize the prediction factors. Results show that the prediction model consisting of 12 forecasting factors, namely, visibility, wind, air pressure, dew point, air temperature, sea temperature, depression of the dew point, air-sea temperature difference, relative humidity, cloud volume, and air-sea 24-h delayed difference—performed best. The threat score (TS) of this model for sea fog prediction was approximately 0.64 in 2018, and the accuracy rate was approximately 0.783. This method shows good performance for sea fog prediction. Moreover, it provides a new approach for the operational forecasting of sea fog. |
Key words: sea fog machine learning ensemble forecast Qingdao shelf |