本文已被:浏览 428次 下载 1061次 |
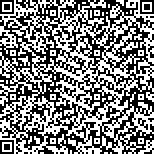 码上扫一扫! |
|
基于点云与影像融合的黄河三角洲互花米草提取方法 |
曹裕超1,2, 王健1, 邵为真3, 孙文潇4, 曾静静5
|
1.山东科技大学 测绘与空间信息学院, 山东 青岛 266590;2.宁波市阿拉图数字科技有限公司, 浙江 宁波 315042;3.山东新汇建设集团有限公司, 山东 东营 257091;4.山东建筑大学测绘地理信息学院, 山东 济南 250101;5.青岛市勘察测绘研究院, 山东 青岛 266032
|
|
摘要: |
针对卫星遥感技术在对滨海湿地互花米草监测时受分辨率、气候条件等多种因素限制存在一定局限性且通过单一的影像数据提取互花米草时精度不稳定的问题,提出了基于无人机点云与影像融合的面向对象互花米草提取方法。以黄河三角洲自然保护区为研究对象,获取了该区域的点云和多光谱影像。先将地面滤波后提取的植被点云与多光谱影像进行特征组合优化,然后对融合影像采用FNEA算法进行多尺度分割后采用基于改进的最近邻算法进行面向对象分类,最终得到的互花米草生产者精度和用户精度分别达到了82.53%和86.43%,较未融合点云的提取精度分别提高了22.34%和7.66%,分类结果的总体精度从89.54%提升至92.61%,且融合点云后影像能够有效区分两种生长状态的互花米草,表明本文提出的方法能够有效提高互花米草的提取精度。 |
关键词: 黄河三角洲 互花米草 无人机 数据融合 面向对象分类 |
DOI:10.11759/hykx20220114001 |
分类号:TP79 |
基金项目:山东省重大科技创新工程项目(2019JZZY020103) |
|
Extraction method of Spartina alterniflora in the Yellow River Delta based on point cloud and image fusion |
CAO Yu-chao1,2, WANG Jian1, SHAO Wei-zhen3, SUN Wen-xiao4, ZENG Jing-jing5
|
1.College of Geodesy and Geomatics, Shandong University of Science and Technology, Qingdao 266590, China;2.Ningbo Alatu Digital Science and Technology Co., Ltd, Ningbo 315042, China;3.Shandong Xinhui Construction Group Limited Company, Dongying 257091, China;4.College of Surveying and Geo-Informatics, Shandong Jianzhu University, Jinan 250101, China;5.Qingdao Institute of Geotechnical Investigation and Surveying Research, Qingdao 266032, China
|
Abstract: |
Considering the limitations of satellite remote sensing technology for monitoring Spartina alterniflora in coastal wetlands, which are affected by factors such as resolution and climate conditions, and the unstable extraction accuracy of S. alterniflora from single-image data, an object-oriented extraction method of S. alterniflora was proposed based on unmanned aerial vehiclepoint cloud and image fusion. The point cloud and multispectral images of the Yellow River Delta Nature Reserve were used as the research subjects. First, the extracted vegetation point cloud after ground filtering was combined with the multispectral image for feature optimization. Then, the fused image was segmented by the fractal net evolution approach algorithm. Finally, object-oriented classification was performed based on an improved nearest neighbor algorithm. The producer and user accuracies of S. alterniflora reached 82.53% and 86.43%, respectively, representing improvements of 22.34% and 7.66% compared to results without point cloud fusion. The overall accuracy of the classification results increased from 89.54% to 92.61%. Therefore, image and point cloud fusion can effectively distinguish between the two growth states of S. alterniflora. This work demonstrates that the proposed method can effectively improve the extraction accuracy of S. alterniflora. |
Key words: Yellow River Delta Spartina alterniflora UAV data fusion object-oriented classification |