|
|
|
本文已被:浏览 362次 下载 473次 |
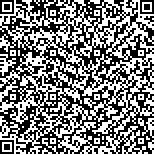 码上扫一扫! |
|
基于梯度提升决策树模型的Sentinel-1图像浅海水深反演 |
黄茂苗1, 魏永亮1,2,3, 唐泽艳4, 刘浩1, 袁文枭5, 袁新哲6
|
1.上海海洋大学 海洋科学学院, 上海 201306;2.上海河口海洋测绘工程技术研究中心, 上海 201306;3.上海海洋大学 国际海洋研究中心, 上海 201306;4.自然资源部 东海发展研究院, 上海 201306;5.西南科技大学 环境与资源学院, 四川 绵阳 621010;6.国家卫星海洋应用中心, 北京 100081
|
|
摘要: |
利用合成孔径雷达(Synthetic Aperture Radar, SAR)反演浅海水深在海洋遥感中极具挑战性。本文采用梯度提升决策树(Gradient Boosting Decision Tree, GBDT)为核心的机器学习算法, 使用Sentinel-1、全球水深数据、风场和流场数据来反演杭州湾和长江口南缘相连的浅海区域的水深。首先分析反演的最佳风速和迭代次数, 再对0~10 m、10~20 m、20~30 m、30~40 m、40~50 m的分段水深和0~10 m、0~20 m、0~30 m、0~40 m、0~50 m的总体水深用相关系数、均方根误差和平均绝对误差进行精度评价, 最后分析反演水深的空间分布特征。结果表明: 反演的最佳风速约为3.78 m/s, 并且GBDT模型达到最佳精度时的迭代次数远小于其他模型, 最佳迭代次数为4。分段水深中, 40 m以内的相关系数都高于0.8, 其中以10~20 m的相关系数最高, 为0.9; 40~50 m则最低, 为0.73。40~50 m的平均绝对误差和均方根误差均为最大, 分别为1.89 m和2.24 m, 20~30 m的平均绝对误差和均方根误差均为最小, 分别为0.75 m和0.96 m。在总体水深中, 虽然随水深区间的扩大, 相关系数会逐渐增加, 但是平均绝对误差和均方根误差的精度都随水深区间的扩大而下降, 且在0~50 m区间内的平均绝对误差和均方根误差最大, 分别为1.06 m和1.59 m, 因此反演的最佳区间为0~40 m。该区域的水深从杭州湾海岸线开始由浅及深阶梯增加, 反演结果能够较好的表现研究区内的实际水深分布情况, 比较符合当前区域的水下地形特征。 |
关键词: 遥感 合成孔径雷达 水深 梯度提升决策树 迭代 |
DOI:10.11759/hykx20221121001 |
分类号:P731.22 |
基金项目:国家自然科学基金项目(41976174;41606196) |
|
Retrieval of shallow water depths from Sentinel-1 images based on a gradient boosting decision tree model |
HUANG Maomiao1, WEI Yongliang1,2,3, TANG Zeyan4, LIU Hao1, YUAN Wenxiao5, YUAN Xinzhe6
|
1.College of Marine Sciences, Shanghai Ocean University, Shanghai 201306, China;2.Engineering Research Center on Estuarine and Oceanographic Mapping, Shanghai Municipal Ocean Bureau, Shanghai 201306, China;3.International Center for Marine Studies, Shanghai Ocean University, Shanghai 201306, China;4.East China Sea Institute for Development Research, Ministry of Natural Resources, Shanghai 201306, China;5.School of Environment and Resource, Southwest University of Science and Technology, Mianyang 621010, China;6.National Satellite Ocean Application Service, Beijing 100081, China
|
Abstract: |
Retrieving shallow water depths using synthetic aperture radar poses significant challenges in the field of ocean remote sensing. In this study, we employed a machine-learning algorithm centered around the gradient boosting decision tree to estimate water depths in shallow sea areas between Hangzhou Bay and the southern margin of the Yangtze Estuary. This was achieved using Sentinel-1 images, global water depth data, and wind and ocean-current data. Initially, we determined the optimal wind speed and number of iterations required for accurate retrieval. The accuracy of these estimates was then assessed across different water depth ranges, namely, 0-10 m, 10-20 m, 20-30 m, 30-40 m, and 40-50 m, for segmented and overall depths of up to 50 m. This evaluation utilized correlation coefficients, root mean square error (RMSE), and mean absolute error (MAE) as the metrics. Finally, the characteristics of the spatial distribution of the retrieved water depths were analyzed. Our findings revealed that the most effective wind speed for retrieval was approximately 3.78 m/s. Notably, the GBDT model required significantly fewer iterations to achieve optimal accuracy than the other models, with the best results obtained after just 4 iterations. In terms of segmented water depth, the correlation coefficients of 0-10 m, 10-20 m, 20-30 m, and 30-40 m exceeded 0.8, with the 10-20-m range showing the highest correlation at 0.9. The 40-50-m range exhibited the largest MAE at 1.89 m and the highest RMSE at 2.24 m, while the 20-30 m range had the lowest MAE and RMSE at 0.75 and 0.96 m, respectively. When analyzing the overall water depths, we observed a gradual increase in the correlation coefficients as the depth range expanded. However, the accuracy, as indicated by the MAE and RMSE, decreased with broader depth intervals. The largest errors were recorded at 0-50 m intervals, with MAE and RMSE values of 1.06 and 1.59 m, respectively, suggesting that an interval of 0-40 m is most reliable for depth retrieval. The water depth in this area gradually increased from shallow to deeper levels, moving away from the coastline of Hangzhou Bay. These results accurately represent the actual water depth distribution within the study area, closely aligning with the underwater terrain characteristics of the region. |
Key words: remote sensing Synthetic Aperture Radar water depth gradient boosting decision tree iteration |
|
|
|
|
|
|