引用本文: | 李海菊,连超,管利聪,李超伦,栾振东.基于R-FCN_ResNet的深海冷泉区生物的识别与分布研究[J].海洋科学,2020,44(9):54-62. |
| |
|
|
|
本文已被:浏览 1103次 下载 1011次 |
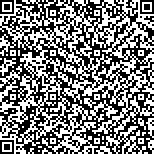 码上扫一扫! |
|
基于R-FCN_ResNet的深海冷泉区生物的识别与分布研究 |
李海菊1, 连超2,3, 管利聪1, 李超伦2,4,5,3, 栾振东6,2,3
|
1.青岛科技大学自动化与电子工程学院, 山东 青岛 266061;2.中国科学院海洋研究所深海中心, 山东 青岛 266071;3.中国科学院海洋大学科研究中心, 山东 青岛 266071;4.中国科学院海洋生态与环境科学重点实验室, 山东 青岛 266071;5.中国科学院大学, 北京 100049;6.中国科学院海洋地质与环境重点实验室, 山东 青岛 266071
|
|
摘要: |
针对深海冷泉生物数量多、密度大、特征低,人工识别它们的种类和数取它们的数量耗时费力且准确度低这一问题,利用残差学习块,改进了基于区域的全卷积网络算法,用以深海冷泉生物的自动识别与检测。首先,构建了一组包括5类目标生物的深海冷泉生物图像目标检测数据集;然后,在TensorFlow框架下对比了R-FCN_ResNet、Faster R-CNN和SSD_MobileNet三种典型的卷积神经网络算法。从训练耗损时间、平均检测速度、平均置信度三方面权衡,突出了R-FCN_ResNet模型的优势;最后,将测试集图片输入至该模型中检测深海冷泉生物的种类和数量,并且以此复现了生物的分布情况。实验证明,所提方法结合本文数据集进行的深海冷泉生物识别与分布研究,是有效可行的,且具有较高的目标分类和定位准确率。 |
关键词: 深度学习 基于区域的全卷积网络 目标检测 深海冷泉生物 |
DOI:10.11759/hykx20191230003 |
分类号:P71;TP181 |
基金项目:中国科学院A类战略性先导科技专项(XDA19060402,XDA22050302);中国科学院重大科技基础设施开放研究项目(NMSTI-KEXUE2017K01) |
|
Recognition and distribution of deep-sea cold-spring organisms based on R-FCN_ResNet |
LI Hai-ju1, LIAN Chao2,3, GUAN Li-cong1, LI Chao-lun2,4,5,3, LUAN Zhen-dong6,2,3
|
1.Institute of Automation and Electronics Engineering, Qingdao University of Science and Technology, Qingdao 266061, China;2.Center of Deep Sea Research, Institute of Oceanology, Chinese Academy of Sciences, Qingdao 266071, China;3.Center for Ocean Mega-Science, Chinese Academy of Sciences, Qingdao 266071, China;4.CAS Key Laboratory of Marine Ecology and Environmental Sciences, Institute of Oceanology, Chinese Academy of Sciences, Qingdao 266071, China;5.University of Chinese Academy of Sciences, Beijing 100049, China;6.CAS Key Laboratory of Marine Geology and Environment, Institute of Oceanology, Chinese Academy of Sciences, Qingdao 266071, China
|
Abstract: |
Because of the large number, high density and low characteristics of deep-sea cold-spring organisms, it is time-consuming, laborious and inaccurate to recognize their species and count their quantity artificially. To solve this problem, this study uses the residual learning block to improve region-based fully convolution network algorithm, which is used for the automatic recognition and detection of deep-sea cold-spring organisms. First, we construct the object detection data set, which should consist of deep-sea cold-spring biological images, and the images contain five kinds of organisms. Then, we compare the performance of three typical convolutional neural network algorithms, namely, R-FCN_ResNet, Faster R-CNN, and SSD_MobileNet, under the TensorFlow framework. The tradeoffs in training time, average detection speed, and average confidence highlight the advantages of the R-FCN_ResNet model. Finally, we input the test set images into the model to detect the species and quantity of deep-sea cold-spring organisms, and based on this, we reproduce the distribution of organisms. The experimental results show that the proposed method combined with the data set in this paper is effective and feasible for the study of recognition and distribution of deep-sea cold-spring organisms, and this means it has high accuracy of object classification and location. |
Key words: deep learning region-based full convolutional network target detection deep-sea cold-spring organisms |
|
|
|
|
|
|