本文已被:浏览 815次 下载 701次 |
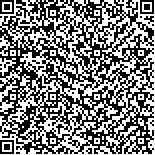 码上扫一扫! |
|
海面太阳耀斑区的溢油光学遥感CNN检测方法研究 |
杜凯1,2, 马毅2,3,4, 姜宗辰1,2, 杨俊芳5
|
1.山东科技大学, 山东 青岛 266590;2.自然资源部第一海洋研究所, 山东 青岛 266061;3.自然资源部海洋遥测技术创新中心, 山东 青岛 266061;4.空天地海一体化大数据应用技术国家工程实验室, 陕西 西安 710072;5.中国石油大学(华东), 山东 青岛 266580
|
|
摘要: |
海面发生大面积溢油事故时,由于太阳耀斑区的存在,海面的油膜在遥感影像上会发生明暗的变化。这对溢油的检测会产生严重的干扰。如何在海面太阳耀斑区准确地检测出溢油是目前溢油检测的难题。针对这一问题,本文利用Landsat7 ETM+多光谱影像数据,开展了基于卷积神经网络(CNN)的海面太阳耀斑区溢油检测方法研究。通过设置对照实验,对比支持向量机、最大似然、随机森林等分类方法,我们发现在相同实验条件下CNN模型的分类精度为95%~99%,Kappa系数为0.92~1,均高于其他三种分类方法,表明了CNN模型在海面太阳耀斑区溢油的检测具有更高的精度与一致性。 |
关键词: 遥感 海面溢油 太阳耀斑区 卷积神经网络(CNN) 分类 |
DOI:10.11759/hykx20200105001 |
分类号:P76 |
基金项目:国家自然科学基金重大项目课题(61890964);山东省联合基金项目(U1906217) |
|
Detection of oil spill in the sun glint region of optical remote sensing data based on the convolutional neural network method |
DU Kai1,2, MA Yi2,3,4, JIANG Zong-chen1,2, YANG Jun-fang5
|
1.Shandong University of Science and Technology, Qingdao 266590, China;2.First Institute of Oceanology, Ministry of Natural Resources, Qingdao 266061, China;3.Technology Innovation Center for Ocean Telemetry, MNR, Qingdao 266061, China;4.National Engineering Laboratory for Integrated Aero-Space-Ground-Ocean Big Data Application Technology, Xi'an 710072, China;5.China University of Petroleum(East China), Qingdao 266580, China
|
Abstract: |
When a large oil spill occurs on the sea surface, it produces a sun glint region that changes the brightness and darkness levels of the optical remote sensing images of the oil spill, which seriously interferes with its classification. Developing a method for the accurate detection of oil spills in the sun glint region is an important problem. Given the urgent need for a solution and the associated practical difficulties, we conducted a study of oil-spill detection methods using Landsat7 ETM+ multi-spectral images in sun glint regions based on the convolutional neural network (CNN). By comparing its performance with that of the support vector machine, maximum likelihood, and random forest classification methods, we found that the CNN model under the same experimental conditions obtained a classification accuracy between 95% and 99%, and a Kappa coefficient of 0.921. These results that were higher than those obtained by the other classification methods prove that for the sun glint regions of oil spills, the CNN has higher classification accuracy and consistency. |
Key words: remote sensing oil spill sun glint region convolutional neural network (CNN) classification |