本文已被:浏览 762次 下载 938次 |
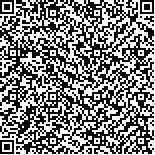 码上扫一扫! |
|
一种增强型YOLOv3的合成孔径雷达(SAR)舰船检测方法 |
张明1,2, 王子龙1, 吕晓琪1,3, 喻大华1, 张宝华1, 李建军1
|
1.内蒙古科技大学 信息工程学院模式识别与智能图像处理重点实验室, 内蒙古 包头 014010;2.大连海事大学 信息科学技术学院, 辽宁 大连 116026;3.内蒙古工业大学, 内蒙古 呼和浩特 010051
|
|
摘要: |
近年来,海战场成为现代战争的主要作战区域之一,舰船目标逐渐成为海上重点监测对象,能否快速准确地识别海战场舰船目标的战术意图,给指挥员的决策提供必要的支持,这关系到一场海上战役的成败。随着合成孔径雷达(synthetic aperture radar,SAR)成像技术的不断发展,大量SAR图像可用于舰船目标检测与识别。利用SAR图像进行舰船目标检测与识别,已经成为重要的海洋应用之一。针对传统SAR图像舰船检测方法准确率较低的问题,本文在YOLOv3的基础上,结合感受野(receptive field block,RFB)模块,提出一种增强型的SAR舰船检测方法。该方法在最近公开的SAR图像舰船检测数据集上平均准确率值达到了91.50%,与原YOLOv3相比提高了0.92%。实验结果充分表明本文提出的算法在SAR舰船的检测中具有较好的检测效果。 |
关键词: 舰船检测 合成孔径雷达(SAR) YOLO 遥感图像 |
DOI:10.11759/hykx20201112001 |
分类号:TP751 |
基金项目:国家自然科学基金项目(61771266,81871430,61663036,62066036);内蒙古自治区高等学校科学研究项目(NJZY18150);内蒙古杰出青年培育基金项目(2018JQ02) |
|
An enhanced YOLOv3 method for synthetic aperture radar (SAR) ship detection |
ZHANG Ming1,2, WANG Zi-long1, LÜ Xiao-qi1,3, YU Da-hua1, ZHANG Bao-hua1, LI Jian-jun1
|
1.Key Laboratory of Pattern Recognition and Intelligent Image Processing, College of Information Engineering, Inner Mongolia University of Science and Technology, Baotou 014010, China;2.School of Information Science and Technology, Dalian Maritime University, Dalian 116026, China;3.Inner Mongolia University of Technology, Hohhot 010051, China
|
Abstract: |
Recently, the naval battlefield has become one of the main operational areas of modern warfare. Ship targets have gradually become the key objects monitored at sea. The success of a maritime battle is related to the ability to quickly and accurately identify the tactical intent of ship targets on the naval battlefield and to provide the necessary support for the commander's decision-making. With the development of synthetic aperture radar (SAR) imaging technology, a large number of SAR images can be used for ship target detection and recognition. Detection and identification of marine targets have become one of the most important marine applications of SAR data. In order to solve the problem of low accuracy of traditional SAR image ship detection, this paper proposes an enhanced SAR ship detection based on the algorithm YOLOv3 and the DarkNet-53 network structure, combined with the receptive field block (RFB) module. This method achieves a mean average precision (Pma) value of 91.50% in the recently published SAR image detection data set and shows an improvement of 0.92% compared with the original YOLOv3. The experimental results show that the algorithm proposed in this paper is effective in enhancing SAR ship detection. |
Key words: ship detection SAR YOLO remote sensing image |