引用本文: | 王蕊,丁咚,李广雪,任昕,韩慧慧.基于改进U-Net的海岸线提取方法[J].海洋科学,2022,46(5):54-64. |
| |
|
|
|
本文已被:浏览 669次 下载 2105次 |
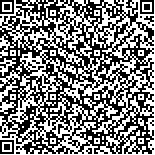 码上扫一扫! |
|
基于改进U-Net的海岸线提取方法 |
王蕊1, 丁咚1,2,3, 李广雪1,2,3, 任昕1, 韩慧慧1
|
1.中国海洋大学 海洋地球科学学院, 山东 青岛 266100;2.中国海洋大学 海底科学与探测技术教育部重点实验室, 山东 青岛 266100;3.青岛海洋科学与技术国家实验室 海洋矿产资源评价与探测技术功能实验室, 山东 青岛 266580
|
|
摘要: |
遥感影像海陆分割对于海岸线提取及其动态监测具有重要意义。传统的基于光谱特征和图像处理的海岸线识别和提取方法, 在面对高分辨率遥感图像复杂的纹理和空间分布时, 只能生成具有局限性的图像特征结果, 且分割结果准确率不高。本文将深度卷积神经网络应用于高分遥感图像的海陆分割问题, 并在经典编码器-解码器结构的基础上进行了创新。首先, 为了降低调参难度引入批归一化层, 降低了网络对参数的尺度和初始值的敏感度; 其次, 采用转置卷积代替传统卷积, 在模型训练过程中通过梯度递减算法, 不断更新参数权值, 显著提高语义分割的精度。利用研究区域高分一号遥感图像数据对于人工岸线及自然岸线的分割实验结果显示: 相较于经典U-Net与SegNet, 改进U-Net网络, 对于各种自然岸线和人工岸线具有更低的边界模糊度和更准确的分割结果, 对于自然岸线的提取结果, 漏检、错检现象较少; 对于人工岸线的提取具有更大的感受野, 能够提取岸线的空间结构信息, 避免误分类。面对日益丰富的高分辨率的遥感影像数据源, 基于改进U-Net的海岸线提取, 能更好地保留边界信息且具备更优的语义分割效果, 可以更为准确地挖掘高分遥感影像的空间分布特征、纹理特征以及光谱特征, 从而提升分类的准确性。 |
关键词: 高分遥感图像 深度学习 海岸线提取 语义分割 U-Net |
DOI:10.11759/hykx20210904001 |
分类号:P737.11 |
基金项目:国家重点研发计划政府间重点专项项目(2017YFE0133500) |
|
Coastline extraction method based on an improved U-Net network |
WANG Rui1, DING Dong1,2,3, LI Guang-xue1,2,3, REN Xin1, HAN Hui-hui1
|
1.College of Marine Geosciences, Ocean University of China, Qingdao 266100, China;2.Key Lab of Submarine Geosciences and Prospecting Techniques (Ocean University of China), Ministry of Education, Qingdao 266100, China;3.Function Experiment of Marine Mineral Resources Evaluation and Detection Technology, Qingdao National Laboratory for Marine Science and Technology, Qingdao 266580, China
|
Abstract: |
Sea–land segmentation of remote sensing images is of considerable significance to coastline extraction and its dynamic monitoring. Faced with the complex texture and spatial distribution of high-resolution remote sensing images, traditional extraction methods based on spectral features and image processing can only generate limited image feature results, and the accuracy of segmentation results is not high. In this paper, a deep convolutional neural network is applied to the sea and land segmentation of high-resolution remote sensing images, and innovation is made based on a classical encoder–decoder structure. First, batch normalization is introduced to reduce the difficulty of parameter tuning, which reduces the sensitivity of the network to the scale and initial value of parameters. Second, transposed convolution is used to replace the traditional convolution. In the process of model training, parameter weights are constantly updated by a gradient decreasing algorithm, which significantly improves the accuracy of semantic segmentation. Segmentation experiment results of artificial and natural shorelines based on Gaofen-1 remote sensing image data in the research area show that compared with the classical U-Net and SegNet, the improving U-Net network has lower boundary ambiguity and more accurate segmentation results for various natural and artificial shorelines. There are fewer adhesions, missed detections, and wrong detection phenomena in the extraction results of natural shorelines. It has a larger receptive field for the extraction of artificial shorelines, which can extract the spatial structure information of the shoreline and avoid misclassification. Faced with abundant high-resolution remote sensing image data sources, coastline extraction based on improving U-Net can retain more boundary information as well as have better semantic segmentation effect and can excavate spatial distribution, texture, and spectral characteristics of high-resolution remote sensing images more accurately, which can improve the accuracy of the classification. |
Key words: high-resolution remote sensing image deep learning coastline extraction semantic segmentation U-Net |
|
|
|
|
|
|